Q1 2024 Research Theme: Gen AI Will Transform the Oil & Gas Sector
- PillarFour Capital Partners
- Mar 31, 2024
- 10 min read
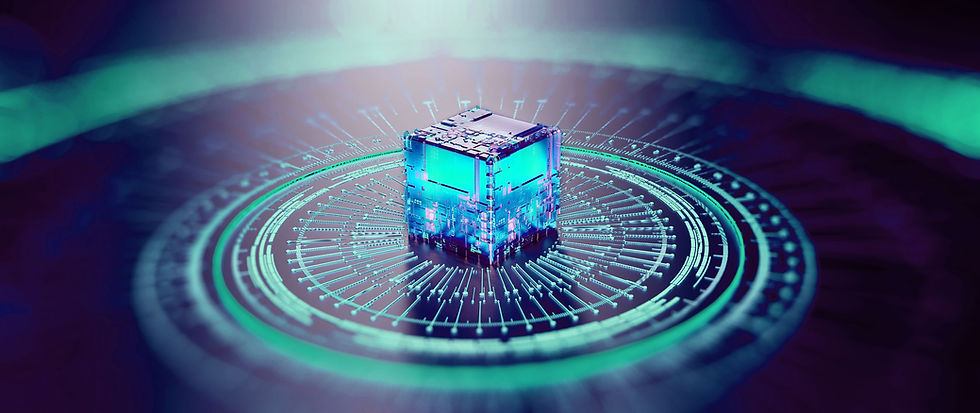
It is almost eighteen months since OpenAI’s ChatGPT product burst onto the scene with a powerful, practical, and consumer-friendly demonstration of AI, in stark contrast to those frustrating AI bots that we too often wrestle with on so-called helplines.
ChatGPT is one of the fastest-growing apps of all time, hosting 1.8 billion visits in March (cf Google hosted 84 billion visits in Dec ’23) despite the raft of competing consumer and professional-focussed AI products that have since entered the market.
Like many ‘overnight’ successes, the foundations of ChatGPT and its many peers lie in advanced deep-learning Generative AI and Large Language models and algorithms, already familiar amongst research and scientific communities for several years.
Generative AI and Large Language Models (Gen AI & LLM to the cognoscenti) have now entered the public lexicon, albeit alongside ‘deepfake’, an inevitable but darker outcome of the rash of Gen AI-driven video and audio apps now available.
Generative AI is an advanced category of AI that can sift through vast amounts of unstructured data - ie information neither available nor stored in a common format – at scale to refine, shape and ultimately generate (hence the name) novel but useful generic patterns and relationships.
Put simply, Gen AI can transform vast, diverse sources of data into tangible insights.
LLMs are a sub-genre of Gen AI, predominantly designed to create human-like textual content.
Big Data and Gen AI enjoy a symbiotic relationship: Gen AI requires a massive array of unstructured data in order to learn and improve decision-making processes; efficient analysis of Big Data can, in turn, usefully leverage Gen AI to discover better, indeed novel, solutions.
The entire resource sector, from oil & gas to metals and mining, is well positioned to benefit from Gen AI:
Operators and service companies alike generate and store a vast array of unstructured data – the lifeblood of Gen AI – across the entire value chain from exploration to production. However, much of this valuable information remains largely untapped, hindering operators’ ability to generate fresh and valuable insights across their scope of operations.
Just consider the variety and scale of unstructured data gathered across the upstream oil & gas sector alone: terabytes of geological, seismic, gravity, drilling and well log data; life-of-field well-by-well production, operational and emission data from downhole, surface and aerial sensors; daily drilling reports, engineering reports, maintenance records, surveillance video et al.
Gen AI offers an efficient route to harnessing the latent power within this ‘ocean’ of data.
The resource sector is well-acquainted with ‘traditional’ AI (i.e. machine learning). Burdened by high capital intensity and complex, often global, operations – but merely commodity pricing for their products – operators have long relied on data analysis to squeeze out costs wherever possible through improved operational efficiencies. ‘Traditional’ AI has therefore been deployed by many companies to refine specific facets of their businesses: e.g. product demand forecasts, predictive maintenance schedules or supply chain management.
Moving beyond ‘traditional’ AI, the surfeit of unstructured data provides a rich opportunity for sifting and analysis via Gen AI to extract and distill complex patterns and relationships within the data, provide novel insights and enhanced predictive models. Furthermore, all such data, insights and models are proprietary in nature – offering a potentially valuable strategic advantage to those companies that decide to exploit Gen AI.
With the growing commercial adoption of Gen AI, the entire oil & gas industry stands at the threshold of a new AI-led horizon that offers the potential for fresh, innovative data-led insights that will improve, even transform, the entire E&P value chain as well as its safety and sustainability.
Here are some illustrative real-life examples of Gen AI-led applications across the oil & gas sector:
Improved Subsurface Imaging, Faster Exploration:
Traditional subsurface imaging – whether seismic, gravity or electromagnetic-based (EM) – is both time and data-intensive, typically requiring terabytes of data, powerful computers and complex physics-based algorithms to analyse and identify exploration targets and relevant subsurface features such as traps, fractures and faults.
Shell and SparkCognition are developing a proprietary Gen AI model to generate reliable subsurface images using far fewer seismic shots. Using advanced image processing techniques, their Gen AI model requires far less seismic data than traditionally required – as little as 1% in field trials! – to generate high-quality seismic images and identify key attributes such as horizons, faults and DHIs – the result being faster exploration at lower cost.
Such Gen AI modelling techniques are likely applicable to other data-intensive subsurface imaging techniques such as airborne full-tensor gradiometry (FTG) gravity and EM.
Improved Reservoir Modelling, Optimal Drilling Strategies:
Gen AI can assist reservoir engineers in building or updating reservoir models by generating realistic, diverse scenarios for key reservoir properties such as porosity, permeability, saturation, pressure and temperature. In turn, improved insights into dynamic reservoir behaviour can refine reserve estimates and optimize well placement and design, ultimately maximising production efficiency and hydrocarbon recovery while reducing emissions.
A leading operator deployed Gen AI to analyse vast amounts of geological, geophysical and operational data including seismic surveys, well logs, and production history to create a detailed reservoir model capable of simulating a complex offshore reservoir. This Gen AI model helped the company identify the most productive drilling locations and optimize well placement, resulting in a 20% increase in oil recovery from the reservoir.
Improved Asset Integrity, Reduced Environmental Risks:
Gen AI can analyse all manner of historic unstructured data – written inspection records, visual inspections, sensor data, cathodic protection data – to retrain and improve the effectiveness of existing predictive-maintenance and corrosion monitoring protocols for vital assets such as pumps, compressors, turbines, process equipment, pipelines et al.
A Gen AI-led maintenance regime offers the prospect of improved throughput, reduced maintenance costs, optimal asset reliability and a reduced risk of environmental pollution.
By way of example, the financial and potential environmental costs of equipment failure are obviously extremely high for those oil and gas majors that operate large-scale offshore production facilities. One supermajor estimated that a mere 1% improvement in their overall offshore platform uptime was worth ca. $300 million annually.
After a successful pilot project, this supermajor rolled out a new Gen AI-led predictive maintenance protocol across two of its offshore platforms – resulting in an initial 4% uplift in platform availability via the avoidance of unnecessary plant shutdowns. Fully deployed across this supermajor’s entire offshore portfolio, the projected economic impact of this new maintenance protocol amounts to about $800 million per annum.
Improved Emissions Monitoring, Reduced Environmental Impact
An international energy company used Gen AI to assess the environmental impact of a proposed offshore drilling project. The AI system gathered and processed a wide array of data, from satellite imagery to local weather patterns and the surrounding marine ecosystem, to predict the potential impact of this drilling project on the surrounding environment. The company believes that the comprehensive array of mitigation strategies that resulted from this Gen AI-led analysis were pivotal in their obtaining relatively swift regulatory approval.
Gen AI can synthesise local meteorological data, emissions data (ground, air or satellite-based) and facility operating data – both real-time and historic – to provide robust early warning of fugitive emissions that exceed regulatory limits, allowing swift corrective action.
Perhaps the grandest example of Gen AI-led emissions monitoring lies with the global initiative jointly led by the Environmental Defence Fund (EDF) and Google.
Successfully launched earlier this year, EDF’s MethaneSAT satellite provides high-resolution coverage of methane emissions from oil and gas facilities worldwide. With both high spatial resolution and precision, MethaneSAT has the capacity to pinpoint small sources of fugitive emissions, currently overlooked by incumbent satellites. Using Gen AI-led quantification and tracking algorithms in conjunction with Google’s AI-led mapping and identification of global oil & gas infrastructure (cf Google Maps), MethaneSAT can accurately and swiftly quantify and track such emissions back to specific oil and gas infrastructure, slashing the lead time from detection to alerting operators and regulators.
MethaneSAT emissions data will, in contrast to other subscription-based emissions data, be published online for free, via Google Earth, providing transparency to all stakeholders and clear accountability for all governmental and corporate emission reduction initiatives.
Optimized Upstream and Downstream Processes:
Gen AI offers the potential to truly optimise upstream completion and production operations – resulting in optimised fraccing programs, lower chemical usage and improved well productivity. Refinery processes such as crude oil distillation or product blending can also be optimised – resulting in lower waste, improved product quality and yields.
Barriers To Entry Diminish As The Unit Cost of Gen AI Collapses
Alphabet, Meta and Microsoft alone are forecast to spend a staggering US$140 billion on Gen AI this year; Amazon likely takes this annual spend up to near US$200 billion.
Given the ‘gold rush’ stampede to establish AI dominance, such expenditures are, somewhat paradoxically, viewed as a badge of honour but are hardly surprising given the sheer amount of data, computing power and energy required to train ever more advanced Gen AI models.
However, while Big Tech continues to commit tens of billions of dollars to Gen AI , the cost to access Gen AI models is collapsing. As OpenAI, Anthropic, Google, Meta, Mistral AI and other AI innovators introduce ever more powerful AI engines, so the ‘unit’ cost of Gen AI for customers is rapidly falling – effectively Moore’s Law on crack!
By way of example, for one food company, year-long access to Gen AI was priced at US$80,000 in October 2023, US$35,000 by January 2024 and currently US$4,000! A legal firm has also seen its Gen AI costs collapse by 80% since October 2023.
As one AI CEO noted: ‘The cost of these [Gen AI] models just keeps falling through the floor’. Another CEO compares Gen AI’s sub-genre of LLMs to ‘electricity’ on the basis that they have become ‘essentially commoditised’.
... Expanding The Potential Benefits Of Gen AI To Smaller E&P And OFS Companies
Gen AI is no longer solely the preserve of well-funded large-cap oil majors or the largest global oilfield service companies. The near ubiquitous availability of Gen AI at low cost will inevitably see more and more smaller companies across the oil & gas sector adopt and likewise benefit from Gen AI-led improvements in productivity and value.
But, as with all products and services that become ubiquitous and commoditised, the future source of competitive advantage for companies will lie in the proprietary data that fuels their AI systems, not the AI or Gen AI system itself.
E&P companies clearly have access to a vast array of proprietary geological, geophysical, drilling, completion and production data. Likewise, oilfield service companies also have access to similar swathes of data, albeit acquired for diverse E&P clients and stratified by type – seismic, gravity, drilling, well log data etc.
The data programming and modelling skills required of AI, let alone GenAI, lie well outside those typical of E&P and OFS companies, but that situation is changing rapidly.
Virtually all major oil and gas companies have been heavily investing in AI companies and technologies to gain operational advantages, enhance safety and meet stringent environmental targets.
By way of example, BP has invested in a variety of AI companies including Grid Edge, Belmont Technologies, Beyond Limits and Satelytics; likewise, Shell has invested in a number of AI companies including Detect Technologies and Quantico Energy Systems, and partnered with SparkCognition, as described earlier.
OFS companies have likewise been investing in both in-house talent and AI companies to maintain, extend or develop fresh competitive advantages. The Big Three all offer open-architecture platforms that allow clients to design, develop and deploy their own AI models at scale.
Baker Hughes partnered with AI company C3.ai back in 2019 and more recently with Corva, a company that delivers AI solutions to clients, primarily related to well construction.
SLB has been working with Gen AI-based LLMs for several years and will continue to advance Gen AI solutions for both in-house and client use. SLB recently partnered with Geminus AI to gain exclusive access to deploy the first Gen AI physics-informed model builder for oil & gas operations. Such Gen AI models provide realistic simulations of physical processes that still conform to the underlying laws of physics – reservoir modelling is just one obvious and valuable application where Gen AI can provide faster, more comprehensive, data-led insights than incumbent solutions.
Conclusion
Gen AI has the power to transform the productivity, economic value, safety and sustainability of the entire oil & gas value chain, ultimately reshaping the industry landscape for both E&P and OFS players.
The next few years will no doubt see Gen AI-led solutions gain further traction and acceptance across the oil & gas sector. Indeed, the race is already underway for both operators and OFS companies as they seek to develop or acquire proprietary Gen AI skills, products and services that will confer an enduring competitive advantage.
What is also abundantly clear is that AI in one form or another – from machine learning to LLMs and broader forms of Gen AI – will become omnipresent across the oil & gas sector, whether for in-house or client-facing applications.
All companies – E&P operators and OFS companies alike – will need to develop AI strategies to remain competitive.
PillarFour is well placed to capitalise on such technological developments within the oilfield service sector given its long-held investment focus on low capital intensity, scalable, proprietary technologies that deliver clear client value.
Download the PDF
This material is intended for information purposes only. This material is based on current public information that we consider reliable, but we do not represent it as accurate or complete, and it should not be relied upon as such. We seek to update our research as appropriate, but various regulations may prevent us from doing so.
Estimates, opinions and recommendations expressed herein constitute judgments as of the date of this research report and are subject to change without notice. PillarFour Capital Partners Inc. does not accept any obligation to update, modify or amend its research or to otherwise notify a recipient of this research in the event that any estimates, opinions and recommendations contained herein change or subsequently become inaccurate or if this research report is subsequently withdrawn.
No part of this material or any research report may be (i) copied, photocopied or duplicated in any form by any means or (ii) redistributed without the prior written consent of PillarFour Capital Partners Inc.
Website links or e-mail communications may contain viruses or other defects, and PillarFour Capital Partners Inc. does not accept liability for any such virus or defect, nor does PillarFour Capital Partners Inc. warrant that e-mail communications are virus or defect free.
This document has been approved under section 21(1) of the FMSA 2000 by PillarFour Securities LLP (“PillarFour”) for communication only to eligible counterparties and professional clients as those terms are defined by the rules of the Financial Conduct Authority. Its contents are not directed at UK retail clients. PillarFour does not provide investment services to retail clients. PillarFour publishes this document as a marketing communication and NOT Independent Research. It has not been prepared in accordance with the regulatory rules relating to independent research, nor is it subject to the prohibition on dealing ahead of the dissemination of investment research. It does not constitute a personal recommendation and does not constitute an offer or a solicitation to buy or sell any security. PillarFour consider this note to be an acceptable minor non-monetary benefit as defined by the FCA which may be received without charge.
This note has been approved by PillarFour Securities LLP (FRN 722816) which is authorised and regulated by the Financial Services Authority.